AI and Machine Learning for Wastewater Treatment Optimization
By Joshua Registe, Senior Data Scientist
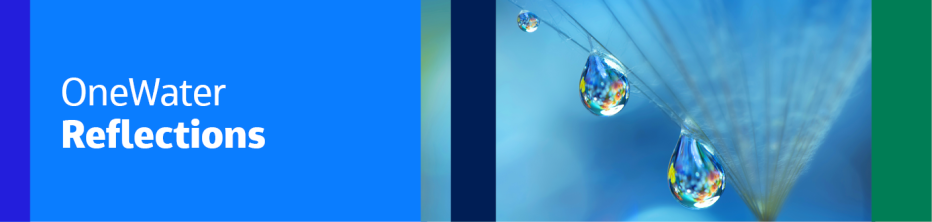
An abundance of data and the rise of artificial intelligence (AI) is creating unique opportunities to optimize water resource recovery facilities.
Wastewater treatment is a highly complex and heavily regulated industry where the priority is to protect public and environmental health. As climate change, supply chain issues and inflation put pressure on energy and chemicals usage at facilities, however, more utilities are looking at ways to safely optimize their wastewater treatment processes. Increasingly, this involves leveraging untapped data sources to unlock efficiencies.
Facilities generate an abundance of data, although the majority goes unused due to the inability of humans to process large amounts of information to support real-time decision-making. AI and machine learning are now making this possible.
I’ll be discussing this topic at WEFTEC, October 5 – 9, where I’ll be presenting two case study applications of Jacobs’ Intelligent O&M solution to optimize chemicals usage at wastewater treatment plants. Using Foundry – a tool by Palantir that enables organizations to integrate, analyze and visualize large and diverse datasets – together with Jacobs’ smart algorithms and subject matter expertise, the solution provides simple real-world recommendations to continually improve operational performance.
One case study is a facility that used excess sodium hypochlorite to treat bacteria, followed by bisulfite to quench the excess chlorine. The addition of bisulfite makes the facility less prone to violations since the concern for high chlorine residuals are mitigated and it allowed the facility to keep dosages relatively high for both chemicals as operators tackle more important challenges at their facility. Using machine learning and modeling data from the facility provides operators with recommendations to optimize setpoints to reduce aeration consumption while maintaining compliance.
At a second similar case study site, a flow-paced sodium hypochlorite system was previously used, typically in excess to capture all events that could lead to high bacterial concentrations. Modeling appropriate dosing profiles with machine learning and probabilistic modeling based on live data has allowed operators to maintain compliance while significantly reducing chemical usage.
Both sites are now regularly achieving anywhere from 10–30% savings in chemical usage. These case studies demonstrate significant progress in the successful implementation of AI at wastewater treatment facilities and the ability to support operators while improving efficiencies.
I’ll be sharing more details on how this was achieved during my presentation at WEFTEC on October 9. Jacobs is also hosting a session featuring Intelligent O&M in the Product Launch Theater on October 7 at 3:30 CT as well as a workshop and networking event on October 8.
About the author
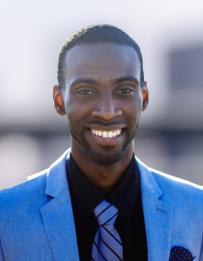
Joshua Registe is a senior data scientist and professional engineer, recognized for his expertise in environmental engineering and advanced analytics. With a unique blend of skills in both domains, he stands at the forefront of cutting-edge AI solution development for Jacobs Intelligent O&M and external clients, tackling intricate environmental challenges head-on. Joshua's contributions span a wide range of critical areas, including solids treatment, disinfection, coagulant optimization, nutrient removal, energy optimization and more. Employing advanced statistical techniques, predictive modeling, deep learning, and scalable computing, he leverages the power of data to deliver unique and complex solutions. In addition to his role as a lead data scientist and professional engineer, Joshua takes pride in mentoring and guiding aspiring professionals in the field. Through his guidance, he fosters the next generation of environmental engineers and data scientists, ensuring the industry's continuous growth and advancement.